Is Your Data Ready for AI?
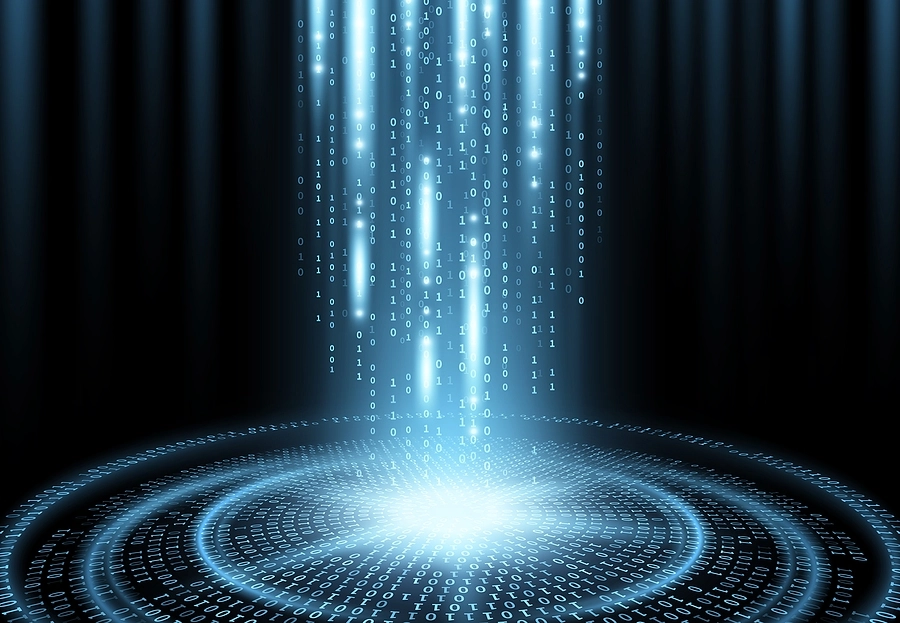
While artificial intelligence has captured our imagination over the past year, it’s also created a new set of problems. Business leaders now must figure out how artificial intelligence—which is a complex and still developing field—will impact their product or service, competitors, people, and business.
AI is likely to be the most transformative technology deployed in generations. Or possibly ever? As of early 2024, the “Skynet Terminator” movie scenario hasn’t happened, and AI has only truly disrupted a few industries so far. Language translation services have disappeared, marketing copywriters have been replaced by smaller teams of editors using ChatGPT, and large language model AI platforms and chatbots have replaced many customer service reps.
The 2023 Hollywood strikes showed that the unions are afraid of AI—digging their heels in to prevent generative AI from replacing writers. When it comes to robots, Cathie Wood, chief executive officer and chief investment officer of Ark Invest, suggested that Amazon could deploy more robots than humans by 2030.
But we still haven’t seen the deployment of the self-driving car, smart contracts haven’t yet replaced escrow services, and accountants still prepare most tax forms. So, it’s a perplexing question for the leadership of most companies outside the Fortune 1000:
How do we deploy an effective AI strategy for our company in such a complex and evolving field?
For the non-technical executive, I’ve found that analogies are effective for translating the complex and abstract into something concrete. But before I share the analogies, let’s share some layperson definitions of AI:
- AI is software that learns and makes decisions, compared to traditional software that executes commands based on predefined rules created by the programmers.
- AI models learn from data patterns and make predictions or decisions without being programmed for each outcome.
AI as the Engine; Data as the Fuel
Now, the analogy that everyone should understand. Think of AI algorithms and models like the engine of a car. Combustion engines convert fuel into energy for motion. This motion moves the car. If you think of AI as an engine, AI software converts data into insights, decisions, or actions. If you give an AI “engine” a burst of data, it can produce an output. If you want continuous output, you must feed it with a continuous flow of data.
The AI “engines” can perform simple automations or complex decision-making processes. Here are examples of simple automations:
- Spelling autocorrection (in iOS and Android text messaging, word editors, and email programs)
- Filtering emails (identifying spam, promotions, or regular emails)
- Netflix or Spotify content recommendations (“Because you watched this, you might like these.”)
- Google / Apple Maps (recommending traffic routes based on traffic sensor data and user-reported incidents)
Here are examples of complex decision-making processes:
- Self-driving cars (analyzing data in real-time from sensors, cameras, radar, and LIDAR to make complex navigational decisions)
- Financial trading algorithms (like BlackRock’s Aladdin, which stands for Asset, Liability, Debt, and Derivative Investment Network—a sophisticated risk platform that executes investment transactions on its own)
- Health diagnostics (analyzing patterns and data from medical images to identify patterns that might not be visible to a human)
- Robotic systems (completing tasks, navigating, interacting with humans or machines)
Just like there is a wide variety of engines, from a simple 2-stroke weed trimmer engine to a Rolls Royce jet engine, there are a variety of AI engines that serve different purposes. Some AI solutions use a combination of these “engines” to produce output.
For your AI strategy, think about a department of your business and contemplate what tasks could be automated by an AI engine. Of course, most of these scenarios involve replacing tasks completed by your people. While history has shown that technology breakthroughs often displace large swaths of workers, those who “did it the old way” learn new skills, get new jobs, and markets evolve. So, I encourage people to think of AI technology as a “co-pilot” to empower a human doing her job, not to replace her. Think of the top 20% of your workers; if you could make your top performers 10x more productive, what would that do to your top and bottom lines?
Data Transformation, or Processing as Refinement
Since data is the fuel for AI, incomplete, biased, or low-quality data can lead to inaccurate decisions or outputs from AI engines—the same as impure gasoline can reduce a combustible engine’s performance.
If you’re using AI for a one-time decision, then data scientists will prepare the dataset and use it once for the output. This work is mostly manual.
If you’re using an AI engine continuously, then you need an automation system to continuously feed data, clean data, structure data, and transform it for delivery to the engine for the output, just like the fuel system injects gasoline in a car. This is an important understanding:
- For a single AI output, a data scientist can acquire and transform the data manually for the engine.
- For continuous AI output, you need to automate your data acquisition and transformation processes (or hire a team of data engineers and data scientists to continuously perform this work).
Maintenance and Optimization
Like automobile and jet engines, AI systems require regular maintenance and tuning to run smoothly. Data inputs and formats change, so maintenance ensures the integrity of the data quality and data transformations to ensure that the outputs remain high-quality and relevant.

As you’re thinking about potential “engines” to automate processes and intelligence in your business, remember that simpler engines require less maintenance than complex engines, so I always recommend starting with simpler automations before moving to the more complex automations.
Start with Your Data
The “engine” and “fuel” analogies are useful for conveying the complexities of AI and data science in an understandable manner. They highlight the importance of both the algorithms that drive AI systems and the data that fuels these algorithms, emphasizing that the quality and preparation of data are as crucial as the sophistication of the AI models themselves.
Our Rehinged AI platform handles the automation of the data acquisition and transformation, enabling our clients to add their own custom “engines” to the platform to deploy continuous AI output for specific use cases within their organization.
We’ve done the heavy lift for those outside the Fortune 1000 who want to leverage the most transformative technology of our generation to “monetize their data” as an asset to deliver intelligence to their people.
Connect with us if you’d like to start a discussion.
Stay connected
Get updates about the Rehinged platform, company news and artificial intelligence.
